Why industrial companies will increasingly rely on machine learning in 2023
Big uncertainties, smaller batches, demographic change: Swiss data science specialist LeanBI explains why industrial companies are becoming more seriously interested in machine learning.
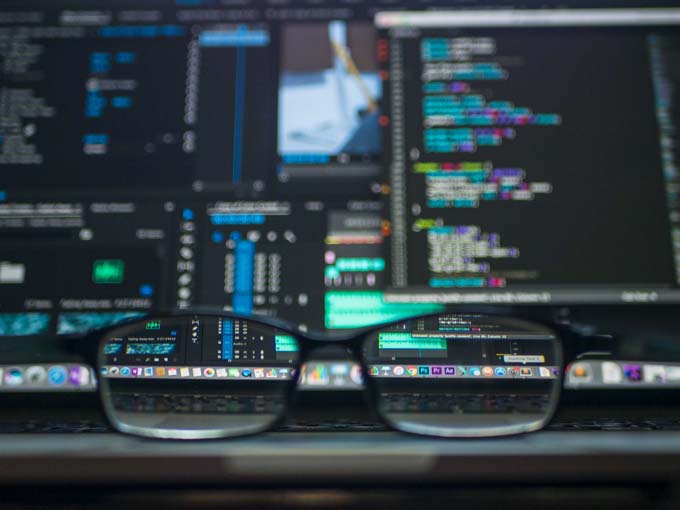
Machine learning has been hyped in the industry in the past. However, the reality has so far fallen short of expectations. Although the technology is certainly being used in production, it is spreading there only with great delay. According to LeanBI, a Swiss specialist for data science applications that develops customized data analysis solutions based on artificial intelligence, this is about to change. There are four main reasons why industrial companies will increasingly rely on machine learning in 2023.
- Volatile environmental factors. The global crises lead to delivery delays for input materials and make sales forecasts more difficult. If industrial companies want to take all influencing factors into account, their corporate planning becomes highly complex. This complexity can only be mastered with the help of machine learning systems. They can significantly help companies to forecast developments while taking a wide variety of scenarios into account - and thus ensure reliable delivery to end customers.
- Individualized production. The batches of industrial companies are becoming smaller and smaller because their customers increasingly expect individual product solutions. To cope with this development, they need to increase the performance of their manufacturing processes. For this reason, industrial companies will increasingly implement predictive maintenance and predictive quality applications. They make it possible to avoid unplanned downtimes and the generation of scrap through timely intervention, thereby optimizing overall equipment effectiveness.
- Energy scarcity and ESG. The current energy shortage will remain the normal state for the foreseeable future. Industrial companies are therefore forced to make their productions as energy-efficient as possible. Machine learning systems enable them to measure and analyze energy consumption online and take it into account in production planning. Collecting energy data also enables them to meet increasing ESG (environment, social, governance) requirements. For example, they can equip their products with environmental and energy labels or provide traceable proof of ESG compliance at any time by historizing the data.
- Demographic change. Workforces are aging, many employees are retiring soon and cannot be adequately replaced due to the shortage of skilled workers. This means that industrial companies are losing valuable expertise in machine management. In many manufacturing processes, influencing factors such as materials are subject to strong fluctuations that cannot be absorbed by a recipe. Machine operators therefore level out these fluctuations through process interventions based on years of experience. To ensure that this know-how is not lost, companies will try to transfer it directly to the machines. Special machine learning approaches based on ontologies such as Bayesian networks are best suited for this.
"Machine learning can provide the best answer to many challenges faced by industrial companies," explains Marc Tesch, owner and CEO of LeanBI. "The conditions are favorable, because in recent years many companies have already worked on equipping their machines with sensors for data acquisition through digital retrofit, networking the machines and bringing the data to the cloud. Now they can take the next step and profitably analyze their data with machine learning algorithms."
Source: LeanBI